A/B testing has become a vital tool for businesses and organizations aiming to improve their offerings through data-driven decisions. However, many fall into the trap of misunderstanding statistical significance, which can lead to incorrect conclusions and ineffective strategies. In this post, we will examine the significance of statistical understanding in A/B testing, clarify its interpretation, and highlight its importance.
What is A/B Testing?
A/B testing compares two versions of a single variable to determine which one performs better in a specific context. This method is widely recognized in areas like website design, email marketing, and product features. For example, if a retailer wants to increase the click-through rate of their email campaigns, they might send one version with a red button and another with a green button to see which color generates more clicks. By randomly assigning users to different versions, A/B testing provides concrete insights into preferences.
Understanding Statistical Significance
Statistical significance helps determine whether the results of a test are likely due to chance. In A/B testing, it evaluates the probability that the observed difference in outcomes between groups is real. When a result is statistically significant, it indicates enough evidence to support that the observed effect is genuine. For instance, if a website redesign results in a 15% increase in user engagement with a p-value of 0.03, it suggests that the redesign likely contributed to this change rather than random fluctuations.
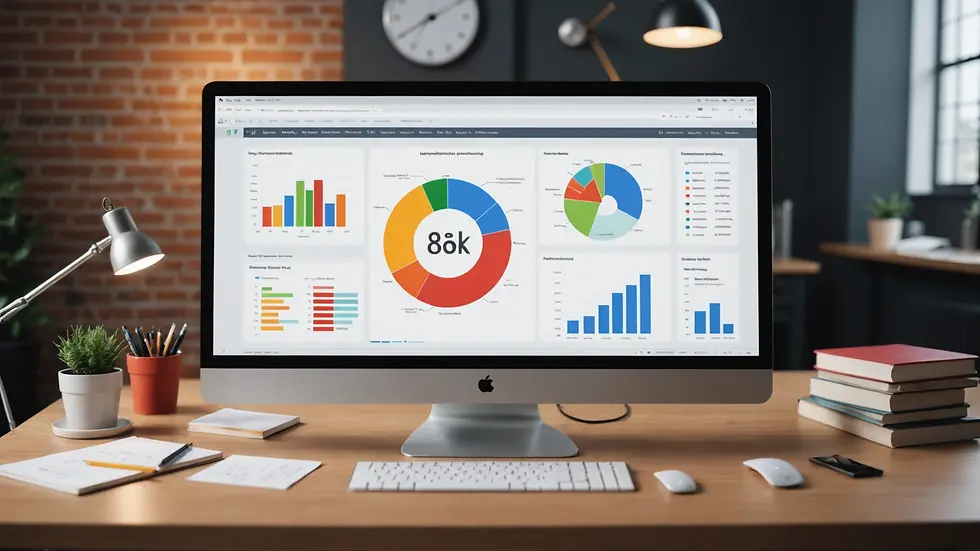
The Importance of Statistical Power
Statistical power indicates the chance of correctly rejecting the null hypothesis when it is indeed false. In A/B testing, high statistical power (typically 80% or more) reduces the risk of Type II errors, which occur when a real effect goes undetected. For example, in a study with 100 participants, a small effect may be overlooked, while another study with 1,000 participants might catch that same effect due to better statistical power. To achieve reliable test results, it's essential to plan A/B tests with enough participants, especially when the effects you are measuring are subtle.
P-Values Explained
P-values are a common metric for determining statistical significance. They indicate the probability of observing the results by chance if the null hypothesis were true. Generally, in scientific fields, a p-value of less than 0.05 is considered significant. However, it's important to remember that this threshold is not absolute and can vary across disciplines. For example, in clinical trials, a stricter threshold of 0.01 may be used.
Interpreting p-values requires care. A low p-value suggests strong evidence against the null hypothesis, while a high p-value indicates weak evidence. It is critical not to view p-values as direct measures of effect size or practical significance.
Confidence Intervals: A Wider Perspective
While p-values serve as useful indicators, confidence intervals provide a broader view of the uncertainty surrounding point estimates. A confidence interval outlines a range of values likely to contain the true effect size at a specified confidence level, usually 95%. For instance, if your confidence interval for the difference between two groups ranges from 3% to 8% and does not include zero, this indicates a statistically significant difference. This additional context helps assess the variability and reliability of observed effects.
Common Pitfalls in Interpreting Statistical Significance
Several common misinterpretations can lead to flawed conclusions in A/B testing:
Misleading P-Values: A p-value below 0.05 does not, in itself, signal a large or meaningful effect. Organizations may hastily implement changes based solely on statistical significance without evaluating practical impact.
Post-Hoc Analysis: Analyzing results after the test can inflate p-values and lead to misleading conclusions. A strict pre-test plan is essential for maintaining integrity.
Neglecting Confounding Variables: External factors can skew results if not accounted for. Careful design and control in A/B testing are crucial for accuracy.
Balancing Statistical and Practical Significance
While statistical significance is valuable, it does not offer the complete picture. Practical significance concerns whether the effect size is meaningful in real-world scenarios. For example, finding that a new feature increases click-through rates by 0.2% might yield statistical significance, but if that change translates to only a handful of additional users, it may not be worth the investment.
For effective decision-making, consider both statistical and practical significance. Business leaders often have to weigh factors like cost and user impact with statistical data. In a real-world case, a company that changes its website layout may find a significant increase in bounce rates, but if the change leads to higher conversion rates, the positive impact outweighs the negatives.
Making Informed Decisions
To leverage statistical significance effectively in A/B testing, consider these strategies:
Set Clear Goals: Define what success means for your test. This clarity will help in choosing the right metric to analyze.
Choose the Right Sample Size: Perform power analysis to determine an adequate sample size based on expected effects and necessary power.
Monitor Results Over Time: Look for trends rather than relying on single test outcomes. Analyzing data over time can provide valuable context.
Communicate Effectively: Clearly differentiate between statistical significance and practical implications when sharing results with stakeholders.
Key Takeaways
Understanding statistical significance is vital for A/B testing. It empowers organizations to make decisions based on evidence rather than guesswork. By grasping key concepts like p-values, confidence intervals, and the distinction between statistical and practical significance, businesses can harness the full potential of their data. As you embark on your next A/B test, keep these principles in mind to ensure valid and insightful results that effectively guide your strategies.
Comments